
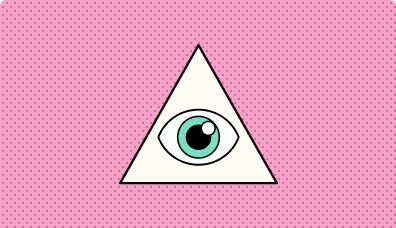

Apache Kafka is a powerful platform for creating distributed event streams - it allows you to read, write, store and process events at scale. However, the extent to which Kafka can do these things well depends on how you configure your Kafka cluster. Kafka has a complex architecture, and the efficiency of Kafka data streams can vary significantly based on how individual components of Kafka are set up, as well as how they integrate with each other.
To provide guidance on getting the best performance from Kafka, this article explains best practices for configuring one key Kafka component: Consumers. Below, you'll learn where Consumers fit within the Kafka architecture, how to set up a Consumer and which Consumer best practices can help to supercharge Kafka clusters.
What is Kafka? Kafka cluster components, explained
Before diving into best practices for working with Kafka Consumers, let's discuss the overall Kafka architecture and the main components of a Kafka cluster.
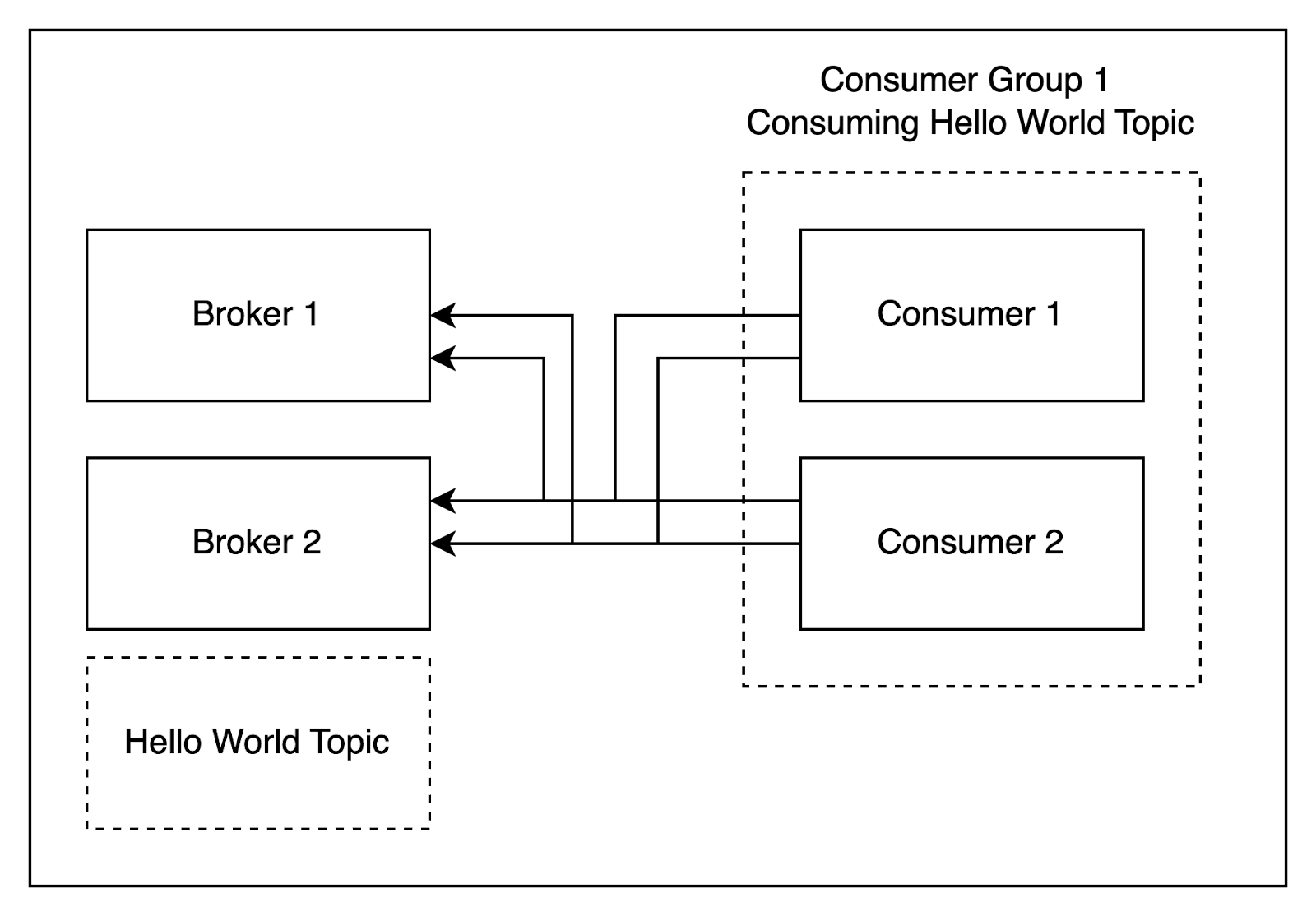
Kafka, as we've said, is a distributed event-streaming platform. Its purpose is to stream data in real time – or as close to real time as possible – so that applications and services that need to send and receive data can do so in an efficient, reliable, continuous and scalable way.
To enable streaming data, Kafka relies on several distinct components:
- Producers: A Producer is an application or other resource that generates data. In other words, Producers are the source of Kafka data streams.
- Brokers: A Broker is a server that runs within a Kafka cluster. Its job is to store Kafka Topics, as well as to save the state of each Consumer that consumes those topics. Kafka's distributed architecture means that you can have multiple Brokers – which is a great thing, because the more Brokers you have, the more you can distribute data streaming tasks across them in order to optimize performance and reliability.
- Topics: Topics are categories of messages. You can organize data streams into Topics to keep track of different types of messages. Topics can be partitioned across multiple Brokers. Every topic is separated into Partitions.
- Partitions: Partitions are sub-components of Kafka Topics. Partitions make it possible to divide Topics into smaller components, which can then be distributed across multiple Brokers that share in the work required to stream the messages associated with each Topic. Every Partition can be consumed by a single Consumer within a consumer group, which means that the maximum number of Consumers determines the maximum number of Partitions you can create.
- Consumers: Last but not least, a Consumer is an application that consumes messages associated with a Kafka Topic from a Broker. In other words, a Consumer is the component on the receiving end of a Kafka data stream. We'll see later in this article, how configuring Consumers can have a dramatic impact on overall Kafka performance.
When you put these components together, you get a Kafka cluster that can stream one or several categories of messages using a distributed architecture.
Key Kafka concepts
In addition to understanding the basic Kafka cluster architecture, it's also useful to understand some key Kafka concepts – Consumer Groups, offsets, lag and replication factor – if you want to optimize the performance of your data stream.
Consumer Groups
A Consumer group is a collection of registered consumers under the same Consumer Group name – such as Consumer Group in the diagram below.
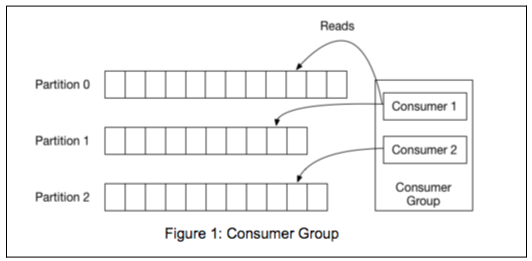
Offsets
Every Consumer Group contains an offset for each Partition. The offset represents the location within the Partition where the Partition's Consumer is currently reading data.
Offsets allow Consumers to resume data consumption at the same spot where they left off, in the event that the Consumer goes down and restarts or any change in the amount of registered Consumers on that specific Consumer Group.
So, offsets are important for ensuring continuity within data streams, as well as for preventing data loss or missed messages during Consumer downtime.
Lag
In Kafka, lag is the number of messages that a consumer has to read before the offset of that partition reaches the end of the partition. Obviously, you want this number to be as small as possible if you're trying to stream data in real time, but there will always be some amount of lag due to issues like network throughput limitations. That said, as we explain below, being smart about the way you manage Kafka Consumers can help to minimize lag.
Replication factor
The replication factor is the number of times a Topic has been replicated across Brokers.
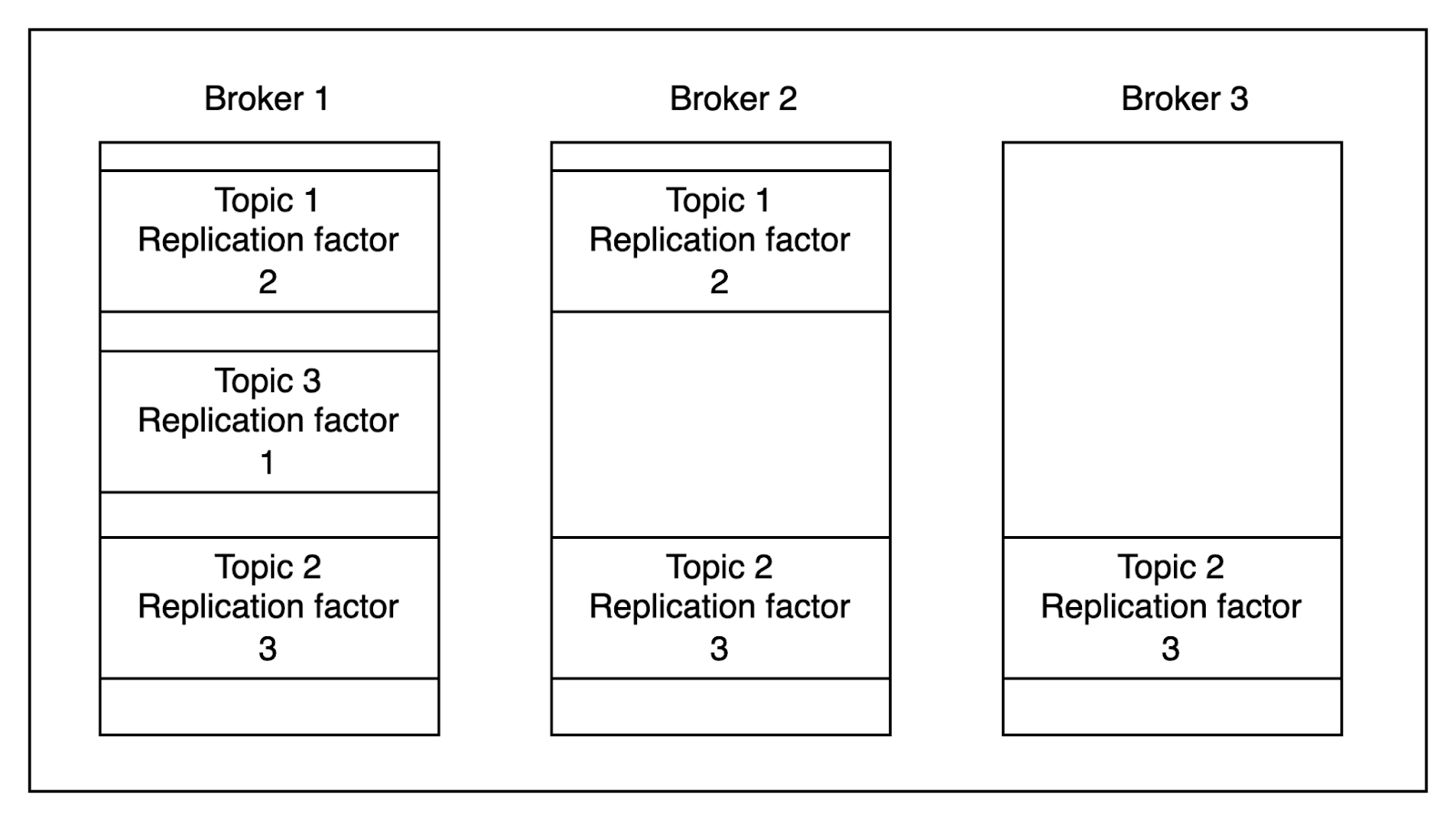
For example, if a Topic has a replication factor of 2, the Topic is being distributed on 2 Brokers. If one Broker were to go down, the Topic would still be available to Consumers because the other Broker would remain up.
The higher your replication factor, then, the greater the reliability of your Kafka data stream.
Creating a Kafka Consumer
We promised to focus on best practices for working with Kafka Consumers, so let's shift our focus to creating Consumers.
Here's an example of a basic Kafka Consumer (borrowed from GitHub):
Sign up for Updates
Keep up with all things cloud-native observability.